REGISTRO DOI: 10.69849/revistaft/ar10202310011040
Fabiana de Sá Ferreira
Abstract
Fuzzy logic has emerged as a groundbreaking tool in medical diagnostics, addressing the complexities and uncertainties inherent in clinical data. Developed by Lotfi Zadeh in the 1960s, fuzzy logic extends classical logic to handle vagueness and imprecision, making it particularly valuable in interpreting diverse and often ambiguous clinical information. Unlike traditional models that rely on precise data, fuzzy logic employs degrees of truth, allowing for a more flexible and realistic analysis of patients’ health conditions. Recent studies have demonstrated the effectiveness of fuzzy logic in enhancing diagnostic processes. Ahmadi et al. (2018) conducted a systematic review that highlighted the significance of fuzzy logic in reducing diagnostic ambiguity and uncertainty, offering insights into its impact on various diseases and identifying under-researched areas. Uzoka et al. (2011) compared fuzzy logic with the fuzzy-analytic hierarchy process (fuzzy-AHP), finding that fuzzy logic slightly outperformed AHP in correlating with conventional diagnoses. Alam et al. (2022) explored the integration of fuzzy inference systems (FIS) with the Internet of Things (IoT) for smart disease diagnosis, showing improvements in accuracy and efficiency for diseases like COVID-19 and malaria. Sudha and Girijamma (2017) introduced an enhanced fuzzy logic algorithm for clustering complex medical data, outperforming traditional methods in response time and classification accuracy. Thomas, Goni, and Emeje (2019) reviewed various fuzzy models, emphasizing their ability to manage nonlinear relationships and uncertainties in medical diagnosis. Shetty (2018) proposed a methodology for predicting heart disease using fuzzy logic, incorporating a web app prototype for wider accessibility. Collectively, these studies highlight fuzzy logic’s potential to improve diagnostic accuracy and efficiency by effectively managing uncertainties and integrating diverse data sources. As technology advances, fuzzy logic is expected to play an increasingly crucial role in enhancing medical diagnostics and patient care.
Keywords: Fuzzy Logic; Medical Diagnostics; Artificial Intelligence; Disease Prediction; Systematic Review.
Fuzzy logic has emerged as a transformative tool in medicine, particularly in diagnosing complex conditions where uncertainty and imprecision are prevalent. Developed by Lotfi Zadeh in the 1960s, fuzzy logic extends classical logic to handle the vagueness inherent in medical data. This approach proves invaluable in interpreting diverse and often imprecise clinical information, facilitating more accurate and personalized diagnoses.
In the realm of medical diagnosis, the imprecision of variables—such as subjective patient-reported symptoms and variable lab results—makes fuzzy logic an ideal tool. Unlike traditional models that depend on precise, well-defined data, fuzzy logic operates with degrees of truth, allowing for more nuanced and realistic analysis of patient health.
Recent advancements in fuzzy logic have enabled better integration and interpretation of various types of clinical data. For instance, fuzzy logic can amalgamate physical exam results, medical histories, and lab findings to provide a comprehensive view of a patient’s condition, which is particularly useful for diagnosing complex diseases with overlapping symptoms, such as autoimmune disorders and rare cancers.
Moreover, fuzzy logic has facilitated the development of decision support systems that aid doctors in evaluating and prioritizing diagnoses based on a range of criteria. These systems use fuzzy rules to account for the nuances and uncertainties in clinical data, enhancing the decision-making process.
Technological advancements in artificial intelligence and machine learning have further enhanced fuzzy logic’s application in medicine. Machine learning models that incorporate fuzzy logic can continuously refine their diagnostic capabilities as new data becomes available, improving the accuracy and reliability of diagnoses over time.
Ahmadi et al. (2018) conducted a systematic review to assess the application of fuzzy logic in medical diagnosis, emphasizing its role in reducing ambiguity and uncertainty. Their study, utilizing the PRISMA methodology and analyzing eight scientific databases, categorized articles based on various criteria such as publication year and medical disciplines. The findings highlighted the effectiveness of fuzzy logic methods in enhancing diagnostic processes and identified areas needing further exploration.
Uzoka et al. (2011) compared the fuzzy-analytic hierarchy process (fuzzy-AHP) and fuzzy logic methodologies in diagnosing diseases, focusing on their handling of imprecise data. Their study found that while neither method showed statistically significant superiority, fuzzy logic had a slightly stronger correlation with conventional diagnosis results compared to AHP, offering valuable insights into the effectiveness of these methodologies.
Alam et al. (2022) demonstrated the use of a fuzzy inference system (FIS) integrated with the Internet of Things (IoT) for smart disease diagnosis. Their system effectively manages uncertainties and provides a robust approach for diagnosing diseases based on symptoms. By employing various coefficients, the system distinguishes between diseases such as COVID-19, Typhoid, Malaria, and Pneumonia, showing that FIS and IoT integration can enhance diagnostic accuracy and efficiency.
Sudha and Girijamma (2017) introduced an enhanced fuzzy logic algorithm for clustering complex medical data, addressing a notable research gap. Their novel algorithm showed superior performance in response time and classification accuracy compared to existing techniques like genetic algorithms and support vector machines, underscoring the effectiveness of advanced fuzzy logic models in medical diagnostics.
Thomas, Goni, and Emeje (2019) reviewed the application of fuzzy logic in handling nonlinear relationships and uncertainties in medical data. They found that fuzzy logic provides a valuable tool for managing the complexities and heterogeneity of various diseases, highlighting its potential to improve the accessibility and efficiency of medical services.
Shetty (2018) proposed a comprehensive methodology using fuzzy logic to predict diseases based on measurable symptoms. The study, applied to heart disease diagnosis, involved fuzzifying symptoms and assigning effect values to represent disease likelihood. This approach, combined with a knowledge base and a web application prototype, aims to enhance accessibility and accuracy in disease prediction.
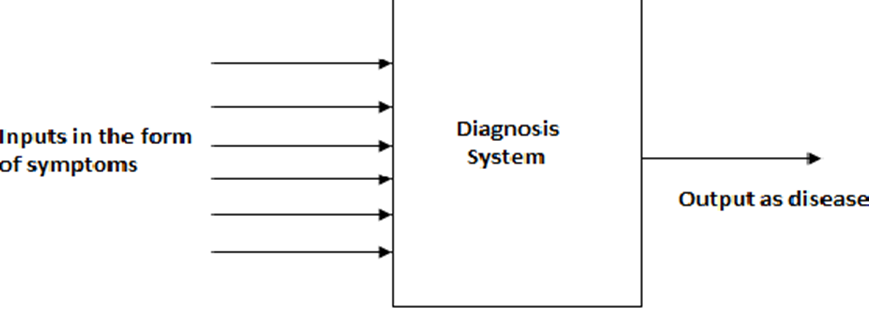
Fuzzy logic has emerged as an innovative and powerful tool in the field of medicine, particularly in the diagnosis of complex and varied conditions. Its application offers several advantages over traditional methods, notably in its ability to handle uncertainties and imprecisions, which are often present in clinical data.
One of the main advantages of fuzzy logic is its capacity to interpret imprecise and subjective data. In medical diagnosis, variables such as patient-reported symptoms and laboratory test results may not be perfectly precise or absolute. Fuzzy logic handles this data flexibly, using degrees of truth rather than rigid categories, allowing for a more adaptive and realistic analysis of patients’ health conditions.
Another significant advantage is fuzzy logic’s ability to integrate and process multiple types of clinical data simultaneously. Instead of relying on just one type of data or test result, fuzzy logic can combine information from physical exams, medical histories, and laboratory results to form a more comprehensive picture of the patient’s health. This is especially useful for diagnosing complex diseases with varied and overlapping symptoms, such as autoimmune disorders or rare cancers.
Additionally, fuzzy logic facilitates the development of decision support systems that assist doctors in evaluating and prioritizing diagnoses. These systems can generate recommendations based on fuzzy rules that account for the nuances and uncertainties of clinical data. By integrating these recommendations with healthcare professionals’ clinical knowledge, fuzzy logic contributes to more informed and precise decision-making.
The advancement of artificial intelligence and machine learning has further enhanced the use of fuzzy logic in medicine. Machine learning models incorporating fuzzy logic can continuously improve their diagnostic capabilities as new data is collected, increasing the accuracy and reliability of diagnoses over time.
In conclusion, fuzzy logic represents a significant advancement in medical diagnostics, offering a robust framework for managing the inherent uncertainties and imprecision of clinical data. By extending classical logic to handle degrees of truth rather than rigid categories, fuzzy logic facilitates a more nuanced analysis of complex health conditions. The integration of fuzzy logic with artificial intelligence, machine learning, and the Internet of Things has further enhanced its applicability, enabling more accurate and personalized diagnoses.
The systematic reviews and studies conducted by Ahmadi et al. (2018), Uzoka et al. (2011), Alam et al. (2022), Sudha and Girijamma (2017), Thomas, Goni, and Emeje (2019), and Shetty (2018) collectively underscore the transformative potential of fuzzy logic in various aspects of medical diagnostics. From improving the handling of imprecise data and integrating diverse clinical information to advancing decision support systems and clustering algorithms, these studies highlight the growing importance of fuzzy logic in modern healthcare.
As technology continues to evolve, the application of fuzzy logic is expected to expand further, providing new opportunities to enhance diagnostic accuracy, efficiency, and accessibility. This evolution promises to contribute to more effective disease management, better patient outcomes, and a more adaptable and precise approach to medical diagnostics, reinforcing fuzzy logic’s role as an essential tool in the healthcare sector.
References
Ahmadi, H., Gholamzadeh, M., Shahmoradi, L., Nilashi, M., & Rashvand, P. (2018). Diseases diagnosis using fuzzy logic methods: A systematic and meta-analysis review. Computer methods and programs in biomedicine, 161, 145-172 . https://doi.org/10.1016/j.cmpb.2018.04.013.
Alam, T., Shaukat, K., Khelifi, A., Khan, W., Raza, H., Idrees, M., Luo, S., & Hameed, I. (2022). Disease Diagnosis System Using IoT Empowered with Fuzzy Inference System. Computers, Materials & Continua. https://doi.org/10.32604/cmc.2022.020344.
Dagar, P., Jatain, A., & Gaur, D. (2015, May). Medical diagnosis system using fuzzy logic toolbox. In International Conference on Computing, Communication & Automation (pp. 193-197). IEEE.
Shetty, R. (2018). Medical Diagnosis using Fuzzy Logic. International Journal for Research in Applied Science and Engineering Technology, 6, 5048-5052. https://doi.org/10.22214/ijraset.2018.4823.
Sudha, V., & Girijamma, H. (2017). Novel clustering of bigger and complex medical data by enhanced fuzzy logic structure. 2017 International Conference on Circuits, Controls, and Communications (CCUBE), 131-135. https://doi.org/10.1109/CCUBE.2017.8394147.
Thomas, L., Goni, I., & Emeje, G. (2019). Fuzzy Models Applied to Medical Diagnosis: A Systematic Review. , 7, 45. https://doi.org/10.11648/J.NET.20190702.15.
Uzoka, F., Obot, O., Barker, K., & Osuji, J. (2011). An experimental comparison of fuzzy logic and analytic hierarchy process for medical decision support systems. Computer methods and programs in biomedicine, 103 1, 10-27 . https://doi.org/10.1016/j.cmpb.2010.06.003.