REGISTRO DOI: 10.5281/zenodo.7985092
Jessica Araujo Sciammarelli
Introduction
There is no longer any doubt that technology is evolving exponentially and that it will be part of people’s lives in all ways, whether at home, at work, in transport and even in moments of entertainment.
Technology has advanced a lot during these years, 20 years ago we never imagined that we would have the facility to access the internet anywhere simply like a minicomputer that is now known as a smartphone.
Currently, there are several new emerging technologies that seek to solve different types of problems, bringing agility and efficiency both in different business models, as well as residential and personal.
It is possible to affirm that within a parameter of a few years, these technologies will mature and evolve more over time.
With the evolution of hardware, allowing for faster processing power and greater memory, Big Data emerged, which is data accumulating in sizes like terabytes, zettabytes, and we currently generate more than 2.5 quintillion bytes daily.
With all this volume and accumulation of data, Data Science and Artificial intelligence has improved, creating an ocean of opportunities for the development and expansion of applications in technology.
As you can see, technology contains a complex cycle, where each technology connects, merges and complements each other in a set.
Even with this evolution and data explosion, the IoT (internet of things) emerged, which are sensors that can be developed for different solutions to solve N problems in different sectors, both in the industrial, residential, logistics, transport and so on. The concept is that these sensors collect data in real time, so this is a perfect storm for data science and artificial intelligence, as they need data for analysis or training, as good data quality is important for their accuracy applications.
Within the concept of Robotics, which was already known in the industrial and factory field, currently gave rise to the context called Industry 4.0, which aims to improve their business models using IoT and especially artificial intelligence to improve the robotics intelligence in developing tasks, these that are getting more and more complex.
These emerging technologies will be approached in a general and introductory context, mainly in the technical part, because to develop at a more technical level it requires more time and more content from the most diverse sources to exercise engineering with responsibility and excellence.
The thesis was divided into modules that are the introduction, description, general analysis, current information, discussions, conclusions, and bibliography at the end.
Description
The content will be divided into stages and discussed separately, through the following context:
1.Big Data
2. Data Science
3. Artificial Intelligence
4.Iot(Internet of Things)
5. Robotics 1.Big Data
Big data is divided into:
Volume – where companies collect data from different locations, which can be IOT, business transactions, etc., with greater storage capacity and this process can be increasingly facilitated. Velocity – data can be collected, stored, processed, and analyzed in real time.
Variety – the data can be of different formats, being classified in structured, unstructured, semistructured in csv, excel, audio, video and so on.
Veracity- which indicates the quality and veracity of the data.
Variability- indicates data variation.
Why is Big Data relevant for companies?
The relevance of Big Data is not in the amount of data and where it is stored, but in the result of the analyzes that can offer valuable insights and answers for companies, such as cost and time reduction, decision making, marketing and so on.
In order to develop several Big Data solutions, you must first define an appropriate strategy of where this data will be collected, where it will be stored, administration and access, so that, finally, the data analysis that will be delivered to the decision makers is obtained.
To analyze big data currently there are multiple sources and ways to do it, to mention some Power BI for Microsoft is a very easy tool that does not require coding.
Taking to the programming playground there are more options such as python and R which are powerful languages that bring high quality solutions in this environment.
Mentioning about decision making, this is the final part, that after the entire process and data analysis, these results that are finalized in reports are delivered to the decision makers who will look and decide the best decision for the future of the organization.
2.Data Science
Before starting any approach related to data science, it is first necessary to define what data science is, and what a data scientist does throughout his career.
Data science emerged due to the explosion of Big Data, generating the storage and processing of a large amount of data from various sources.
The professional data scientist must not only understand programming, but must understand primarily the business model, statistics, machine learning and mathematics, where you can solve problems and get answers in different ways.
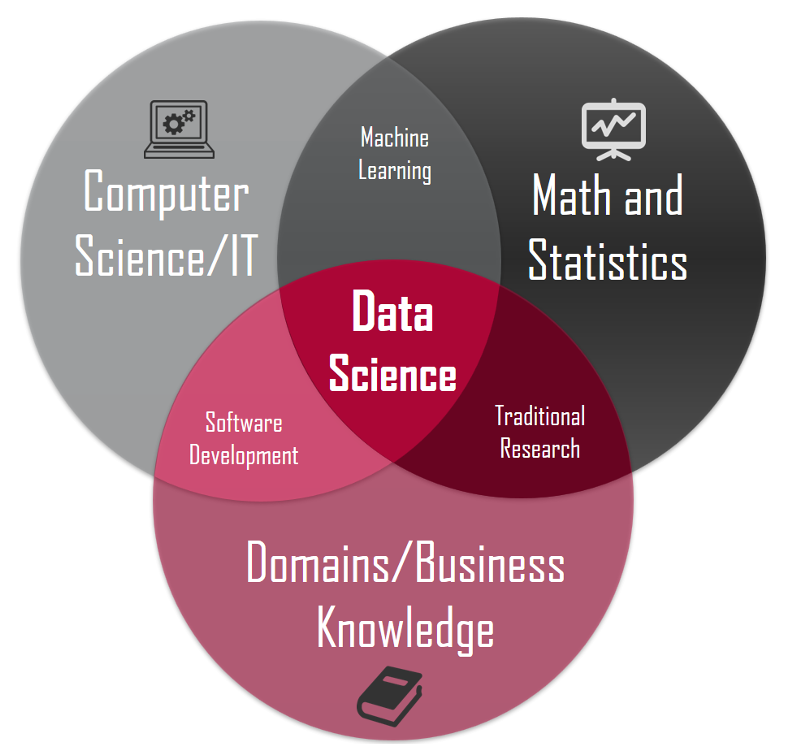
Fig1. Available at https://medium.com/somos-tera/como-migrar-para-ciencia-de-dados-5bef54462419
It was mentioned that with Big Data there was a large amount of data available from different formats and sources.
Well, this is the main raw material in the world of data science, where some people dare to say that data will be the new oil.
This, if the beginning of a cycle of Big Data, data science begins, where first a problem is defined to be analyzed, treated, and solved. Once this concept is very clear and defined, the data that must be collected can be of different formats such as structured, semi-structured, unstructured, located in data warehouses, data lakes or even social networks.
With these data collected, there is still a need to clean them to qualify and analyze them with perfection, if the data is not properly processed, the final result will not be accurate and may cause errors, this is one of the most important and critical throughout the process.
After ensuring that the data has been properly cleaned and treated, the analysis can then be started using machine learning techniques such as regression, classification, supervised and unsupervised models to obtain insights.
When the visualization and data analysis is concluded, a report can then be made, where it must express in a non-technical way about a technical subject for senior management, where often they are not technical people but are responsible for the decisions of the organization, so the importance of the report is made in an objective and not technical way.
3.Artificial Intelligence
Artificial intelligence is the machine’s ability to learn and perform tasks that only then humans are capable of performing.
The machines are able to recognize patterns based on training where a specific database for certain problems is used.
AI generates a lot of controversial issues, where they mention that machines will take the work of humans, and it is important to emphasize that currently with the technologies that we have, it is not possible to develop something related of this type.
However, AI is revolutionizing business models and the way we live, but some jobs can be replaced by machines, jobs that have repetitive tasks, so that humans have more free time to focus on jobs that require decisions, creativity and intellect.
The main AI development activities are classified as:
• Machine Learning
• Deep Learning
• Robotic Process Automation
• Virtual Assistants (Chatbots)
• Computer vision
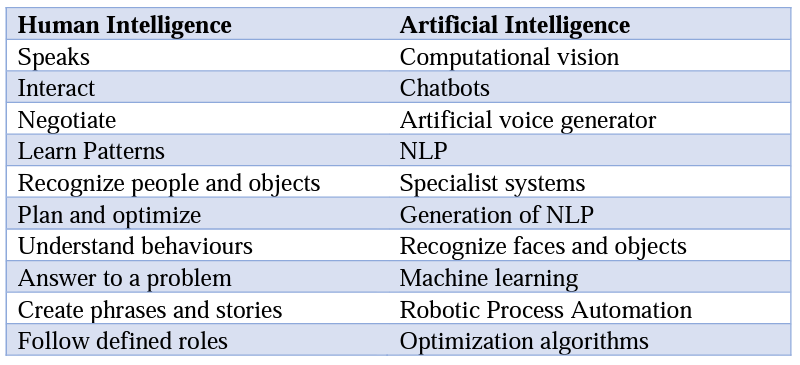
Digging a little deeper, machine learning can be classified into three levels.
Supervised – The algorithms of this learning format are capable of learning. behaviors and patterns associated with each of the classes in the labeled column, Thus, they are able to predict unknown results.
Unsupervised – In this type of learning, problems that are known can be addressed with little or no prior knowledge about how the results could be seen. Therefore, it is not known how each variable of the problem can influence the results.
Reinforcement learning – the focus is on taking appropriate measures in a given situation in order to maximize the reward, that is, this method is used to find the best behavior or path to follow.
This learning differs from supervised because it does not have a correct answer in its training. Instead, the reinforcement agent decides what to do to execute the past task, so in the absence of a training set, he learns through the experience gained in the process, using positive and negative reinforcements, where each imposes its influence differently.
Illustration of Artificial Intelligence and its divisions
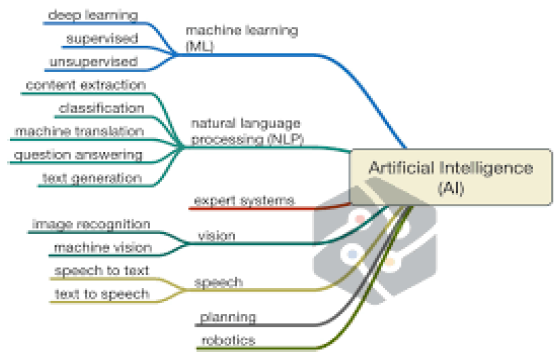
4.IoT(Internet of Things)
IoT, internet of things, as the name implies, is the definition of N “things” that can be created to generate data and transfer it in real time for analysis.
IoT can be used from factories to control their processes or when a machine must perform maintenance, or even digital watches for physical activities, it can be used in the automotive sector to control temperature, home automation control, that is, there is an ocean of possibilities that can be developed from the concept of the internet of things.
IoT is web-enabled smart devices that use embedded systems, such as processors, sensors and communication hardware, to collect, send and act on data they acquire from their environments.
It enables people to live and work smarter, reduce costs by automating tasks and offering more efficiency in its processes.
As nothing is perfect in the world, there are advantages and disadvantages in the process to develop the IoT, to mention some:
Advantages
-Cost reduces.
-Ability to access information from anywhere at any time on any device.
-Improved communication between connected electronic devices.
Disadvantages
-As the number of connected devices increases and more information is shared between devices, the potential that a hacker could steal confidential information also increases.
The most concerning problem in IoT development is the security issues, since all this data becomes available, it can become an easy target for hackers to have access to it. There are some solutions that can fix this issue, which can be cryptography and by hiring cybersecurity experts to monitor and give appropriate care of these sensors.
There is also a technology called Blockchain which is an innovative and emerging solution that can be applied to IoT architecture, but that technology is out of our scope here.
Illustration of the possibilities that can be done with IoT:
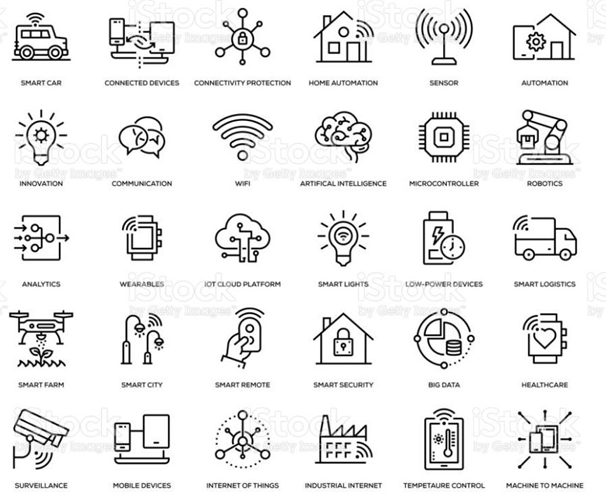
Fig.2 Available at https://www.istockphoto.com/br/vetor/jogo-do-%C3%ADcone-do-internet-das-coisasgm1132960700-300551961
5.Robotics
The concept of robotics leaves the scope within the world of computer science. But due to the cycle of each subject that connects to each other, robotics should also be mentioned, but superficially, since this subject belongs to mechatronics and robotics engineering. However, there is still the participation of computer scientists in the development of these machines, as in almost all technology and engineering the use of programming is required.
Robotics, as the name says, is the production of machines and robots that are personalized to perform different tasks, therefore they are gaining much intelligence as before, because of the improvement of the big data that it takes artificial intelligence to improve as well.
The most known robots are the ones that perform critical tasks in the factory as robot arms. There has been impressive progress in robot humanoids such as Sophia and in particular the many types of robots that Boston Dynamics developed.
Most common use cases of Robots
The robots are found to currently perform diverse tasks in many fields such as:
-Robot arms, the ones that are known to be used in the manufacturing sectors.
-Surgical assistants (a robot that is being manipulated by a doctor to perform a surgery) can be named Doctor Robot as well.
-At home it is possible to see the robot vacuum cleaner
-In the logistics sector by performing tasks such as taking and transporting items
-During the covid 19 it was possible to see more robotics development such as drones to deliver medicine and items, robots in the hospital to deliver food, medicine and items to the patients.
The research and development are not limited to these creations and probably in the future it may appear more and more robotics solutions in the market.
General Analysis
This part of the thesis aims to define the main tools available in the market to work which each emerging technology classified here.
The purpose is not to write codes and go into details, since it’s a general definition of the main 5 potential technologies that are going to play an important role now and so on.
1.Big Data
There are dozens of technologies related to applying Big Data, but it will only be defined five: Hadoop, Apache Storm, Mongo DB, Cassandra and Cloudera.
Hadoop
Known also as Apache Hadoop, is a framework that allows distributed processing or large data sets across clusters of computers using single programming models.
More than all that, it is an open-source software that is reliable, scalable and offers to be distributed computer.
Apache Hadoop has many other software under its umbrella and documentations defining how each one it words.
Benefits of Apache Hadoop:
Scalability – data can be stored, managed, processed and analyzed at petabyte scale.
Reliability – by working with data distribution in clusters, even in times of failure, it continues to work and in a state of recovery.
Flexibility – data can be stored in any structure as well as unstructured and structured.
Low cost – because it is open source, it is a low-cost software.
Hadoop Utilities:
Data Storage – Offers scalable, fault-tolerant storage that spans large clusters of servers and thousands of servers.
Data processing – within the Apache Hadoop framework there are other software that help in this step, mentioning for example MapReduce.
Data analysis – the stored data can be accessed for analysis through the framework’s own software.
Data governance and security – the framework provides software to integrate and increase security in its use.
Apache Storm
It is an open-source system provided by the Apache Foundation; it has the capacity to process more than a million tuples per second in each node. This speed is just interesting for working with ‘streaming’ data (real-time data).
Therefore, it is used in real-time distributed computing, facilitating the process of unlimited data flow.
Even though it is an open-source project, it has been used and appreciated by large companies helping with the Big Data infrastructure. Companies like Yahoo, Twitter have already integrated the framework as part of their processes.
MongoDB
MongoDB is classified as a NoSQL database, the best known are MongoDB, Hadoop, Cassandra among others.
MongoDB is a document-oriented database, unlike traditional databases that are relational model-oriented.
It is an open-source database and was written in C++ language.
Because it is document-oriented, data can be nested in complex hierarchies and still be indexable and easy to search.
It was created precisely to deal with Big Data. It supports both horizontal and vertical scaling using replica sets and shading, being used efficiently especially in unstructured data.
Apache Cassandra
Similar to MongoDB, it is an open-source NoSQL database, which was developed by Facebook, and widely used in large technology companies.
Advantages of using Cassandra
● High availability.
● Performance.
● Extremely fault tolerant.
● Linear scalability: if the bank serves 100K of requests, to meet 200K it is enough to double the infrastructure.
● No single point of failure.
● Highly distributed.
● Supports IN data centers natively.
Cloudera
It offers several solutions in Big Data, providing a platform for the management, monitoring and analysis of large data sets in the most different formats, with a high flow of data.
Currently, there is a greater amount of unstructured data available than structured data, so the importance of having a tool to assist in this process is of great relevance.
It also provides the core elements of Hadoop and is Apache-licensed software.
Data Science R – Programming Language
R was created in the 70s, focusing especially on exploratory data analysis and statistics.
Often used for medium-sized data manipulation, statistical analysis, and document production and data-centric presentation.
R has a typed language, that is, data can be modified even if contained in variables that are already running.
Python
It is a high-level programming language, dynamically typed and strong, with an extensive library and frameworks developed by the community.
Example:
name = input(‘What’s your name?’)
print (‘Hello, %s.’ % name)
Due to its high level and simple syntax, its code is generally more readable and easier to understand compared to other languages like Java, C and C++.
It is very versatile and of wide use, being able to be developed in the most different types of projects, being able to be used in the area of mathematics or even as in projects of artificial intelligence and machine learning.
Excel
Well known in the market, it was created by Microsoft. It has a huge variety of features, functions, and formulas. It is good software to control and organize information.
Within the tasks of a company, tasks such as:
– Revenues.
– Production. – Sales (or services); – Inventory.
– Suppliers.
– Customers.
– Employees and payroll.
– Goals and projections.
Being of great relevance for professionals from different sectors, it can be used to generate reports, tables, graphs, lists, controls etc.
It still has the advantage of being imported into other software such as Power BI for example.
Apache Spark
It is an open-source Big Data tool, with the objective of processing large data sets in a parallel and distributed way.
Spark outperforms Hadoop and can process data 100x faster.
It allows programming in Java, Scala and Python, another notable advantage is that its components can be integrated in the tool itself, unlike the Hadoop Framework.
Having several components, the best known are:
-Spark Streaming, which enables the processing of streams in real time.
-The GraphX, which performs processing on graphs.
-SparkSQL for using SQL in performing queries and processing data in Spark.
-The MLlib, which is the machine learning library, with different algorithms for the most diverse activities, such as clustering.
Tableau
Widely used tool in the field of business intelligence and data analysis. It was created based on the idea of integrating data analysis and reporting. Thus, facilitating the process of the data scientist. It is an easy-to-use application, where anyone with some prior knowledge of excel can quickly learn. It has a scalable architecture, with compatibility for cell phones, web and desktop, being an enterprise level platform.
3.Artificial Intelligence Weka
Weka is an open-source project developed by the University of Waikato, it was developed in the Java language, aiming to perform data mining with great ease and having a friendly interface.
Within the context of artificial intelligence is considered one of the easiest software to work in the field, including beginners in the field.
It is a set of machine learning algorithms to perform data mining tasks, it also contains tools for data pre-processing, classification, regression, clustering, association, and visualization rules, thus being a complete solution for data mining, with the possibility of processing large amounts of data with good performance.
To use Weka, just load the data and select the algorithms determined to the type of problem you want to analyse and then obtain visualization and performance of the selected algorithm.
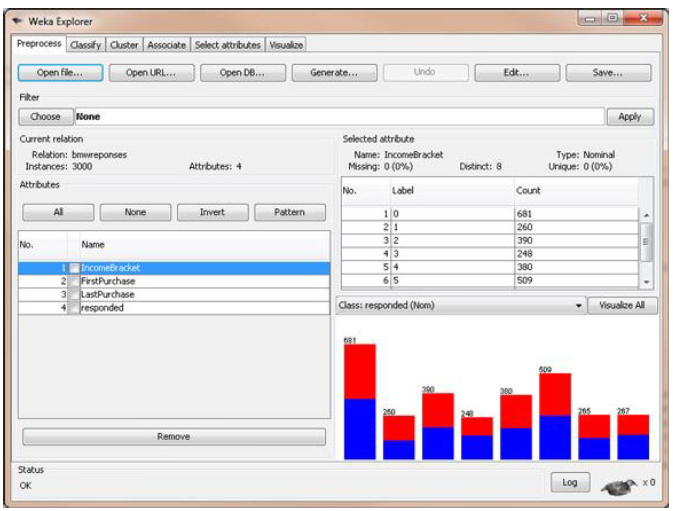
Fig3. Available at https://machinelearningmastery.com/tour-weka-machine-learning-workbench/
Orange
Open-source software works similarly to Weka, however with an even friendlier and easier to use interface, it performs data mining tasks.
Weka and Orange are the easiest tools to use in the field of artificial intelligence as they do not require a great understanding of programming to execute machine learning algorithms.
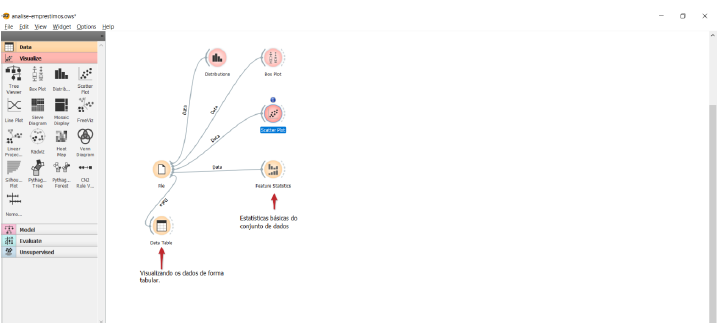
Fig4. Available at https://orangedatamining.com/getting-started/
As illustrated above, it is possible to assemble different types of projects and visualize their results.It features association algorithms, supervised and unsupervised models, predictions and many more.
To start basically only it has to select the dataset and download into it, then choose the appropriate algorithm model for a given problem defined previously, and finally perform the data visualization.There is the option to test several models in the same project and make comparisons between them.
Tensor Flow
Tensorflow was developed by Google and is open source, being widely used in artificial intelligence solutions.Large companies use software, such as Intel, GE, Twitter, Paypal among others.It can run on any operating system, it can also run on CPU, GPU and TPU.
It is easy to use, as there are APIs in several programming languages such as python, C++, Julia, Rust, Scala, C#, Swift, JavaScript among others.
Tensorflow is a framework that is a code union aimed at an application, its programming is very similar to python.
Sample code within the framework:
import tensorflow as tf
phrase = tf.constant(“Hello world!”)
with tf.Session() as sessions:
run = sess.run(phrase)
print(run)
From this concept it is possible to create a neural network using Tensorflow, for example:
w1 = tf.Variable(tf.random_normal([n_input, n_hidden_1]))
b1 = tf.Variable(tf.random_normal([n_hidden_1]))
# Applying the sigmoid function to layer 1
layer_1 = tf.nn.sigmoid(tf.add(tf.matmul(x,w1),b1))
There are other frameworks available such as tensorflow that are used in the work of artificial intelligence, some are open source and others are private that require payment to be used, some examples of other well-known options are Pytorch,Caffe,Theano,CNTK and Keras.
Pytorch
It is an open-source machine learning library specialized in GPU acceleration, automatic differentiation, and tensor computations. Currently it is one of the most popular deep learning libraries and has a competitor such as Keras and Tensorflow.
There are some recommendations regarding the different available frameworks such as:
1.Pytorch is interesting for research and development
2.Keras is the most indicated for beginners
3.For developer’s industries to build applications, Keras and Tensorflow are good choices
Keras
It is an open-source framework that provides a library of neural networks through recurrent and convolutional networks.
It has deep learning solutions with features such as modularity, neural beds, module extensibility and supports Python coding.
It runs on the top of tensorflow background, has easy construction and visualization of deep learning and neural network.
CNTK
It was developed by Microsoft, and there is an open-source deep learning toolkit available for windows and Linux.
It features powerful computation graphs for training and evaluating deep neural networks. Still, it supports feed forward, convolutional, and recurrent networks for speech, image, and text can be used in combinations as well.
CNTK can scales to multiple GPU servers with efficiency and can be included as a library with Python, C++, or C# programming languages, or CNTK model evaluation functionality from Java programs still can.
4.Internet of Things (IoT)
Within the scope of IoT there are several ways to develop these sensors, whether in the hardware or software part, there are other items within the context, but some of the main ones of the present time will be mentioned.
Blockchain
It is a system that excellently tracks the sending and receiving of some types of information over the internet.
It became known worldwide in its use case in Bitcoin and cryptocurrencies, however its technological concept can be widely used in various business models and technological solutions.
A blockchain is a block containing hashing and encryption, ensuring that your information is not tampered with, altered or hacked.
Each block contains its own hash and that of the previous block, thus creating a connection and chain between the blocks.
It has relevance in the development of IoT, as it can solve one of the main problems of the technology, which would be the security issue, since these sensors are exposed in different ways, so using Blockchain can guarantee this layer that was missing for IoT to enter the market with more agility and confidence.
Arduino
Arduino is an open-source prototyping platform, that is, prototypes are carried out through it in order to detect possible flaws and the qualities of the project. It is a small computer that can be programmed to perform a certain function.
As a system that can interact through hardware and software, it can be connected to the network and receive and send data.
The arduino has several applications and can be used in IoT and wearables applications, there are also several types such as arduino uno, arduino pro mini, arduino due among others.
Being open source and its programming language is made in C and C++ language.
Raspberry
The Raspberry is a minicomputer, but the difference is that it has the main components of a computer on a small board.
The device was created in the UK, to encourage education in programming for young people of choice, with an affordable product. Currently there are several models available such as Raspberry PI zero among others.
The device has all the functionality of a computer, so it has CPU, RAM, USB and HDMI
Its greatest use is to build home computers, being connected to a monitor, keyboard, and mouse, it obtains the same functionality as a normal computer, but with a certain limitation, as it is more focused on performing basic tasks such as controlling a home robot or automation of everyday tasks.
5. Robotics
Robotics, as it is the physical part of the agent, does not cover the world of computer science, that is, it goes outside the scope of the area, so it will not be discussed in detail.
However, it is very important to introduce another technical concept that is called RPA (robotic process automation) and its most popular software today.
RPA is easy to interact with, being able to automate simple and repetitive processes, interacting in digital systems to perform operational activities, using the same user interface and interacting with systems.
Its main tasks can be repetitive and manual activities, high volume of data and different applications, tasks that demand a lot of time from scientists.
Automation Anywhere
It is one of the best-known companies in the RPA market, with closed code, that is, it is a software that needs to be paid to use its license.
Being compatible with different segments of companies where they need to automate their business and IT processes,
It is software used to create bots that are automated to manage front and back-office tasks, working in different production environments and offering high levels of security and control.
UiPath
Another well-known company in the RPA market, also working with closed code and offers automation of business and IT processes for companies from the most diverse segments.
Your tool is composed of a studio where the development environment is with pre-programmed functions.
The Robot tool is responsible for executing the automation developed in the Studio process and finally the Orchestrator, which as its name defines, is responsible for realizing the orchestra and harmony of the software as a whole, being responsible for managing the entire automation process.
Its main features are its friendly interface, it does not need experience to use and perform web, process, and remote automation.
Current Information
This topic aims to share what are the new trends, the companies that are making difference with new products and applications.
Big Data
There are new jobs appearing in the market because of the big data effect. Employees need to learn the new technologies and this is a good time for the people that are looking to get into a new career.
Some of the new jobs related to that are Data Engineer, which is the person responsible for collecting all the data and organizing it into a data warehouse or a data lake, so it is ready for the data scientist to utilize it and analyze it.
Some Universities and MOOC have started to offer post graduations and Professional Certificates online, so that it can reach everyone around the world easily.
Since it is a new area in the market still there is not much content available as matured technologies such as cloud computing.
BIG DATA COMPANIES TO KNOW
- IBM
- Salesforce
- Alteryx
- Klaviyo
- Cloudera
- Segment
- Crunchbase
- Oracle
- VMware
- Unacast
- CB Insights
- Databricks
- UserTesting
The giant technology companies are looking into solutions about how to store, process, analyze and maintain all the data that is collected every day and that is getting bigger day by day. Some Companies are developing revolutionary applications that aim to change and improve people’s lives.
There are startups that are bringing new solutions to the market and having high knowledge and visionary people that are looking to advance society by providing innovative products.
IBM has contributed by developing the Hadoop System which is a storage platform that can store all types of data such as structured and structured, it is developed to deal with a large volume of data, so it fills the purpose of the big data. Also has created Stream Computing that enables organizations to perform analytics in real time data processing.
Amazon is another giant tech company that is very known for their SAAS (software as a service) products specially related to cloud computing. That it aims the data to be collected, stored, processed, visualized and analyzed by organizations.
Nevertheless, it is not different for Google and Microsoft, proven giant tech companies in the market who provide innovative services and products related to Big Data and all its infrastructure.
Data Science
Data Science is not different from Big Data, since both are complementary, data is the fuel of DS , there are new jobs related to the subject which is the Data Scientist or Data Analyst, the name is similar but the jobs responsibilities are a bit different.
Data Scientist deals with all types of data, has to be able to understand programming, math’s, machine learning and statistics so it can provide predictive solutions to the organizations.
On the other side, the Data Analyst has to deal with the data also, but only with structured data, probably working with excel, power BI so it can extract insights from historic data, but it is not making predictions as the data scientists.
Illustration that it classify and explain the difference between the jobs
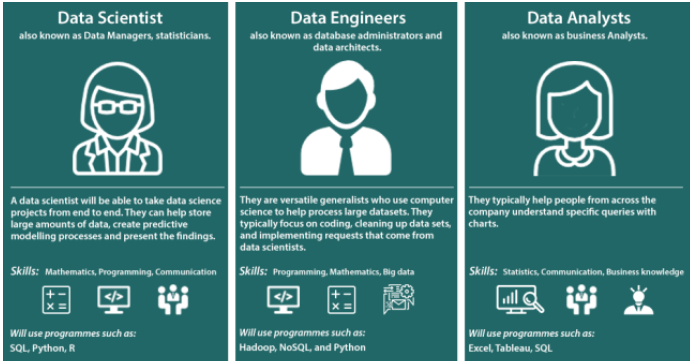
Fig.5 Available at : https://revolucaofeminina.com.br/2020/07/14/ciencia-de-dados-introducao.html
To work in the positions above the education it can be from multiple sources. Mathematicians, economists, engineers, statisticians, and computer scientists can start a career in the field of data science. Therefore, there are available under graduation, post graduations and even master programs related to the subject. MOOC is available as well on a large scale.
Not only the technology companies, but every organization is looking into the type of this employer since it brings competitive strategy and support in higher quality of decision-making process.
3.Artificial Intelligence
AI is also getting benefits from the Big Data environment and new job roles and responsibilities have been appeared such as AI Specialist, AI Machine Learning engineer and AI Researcher Scientist .
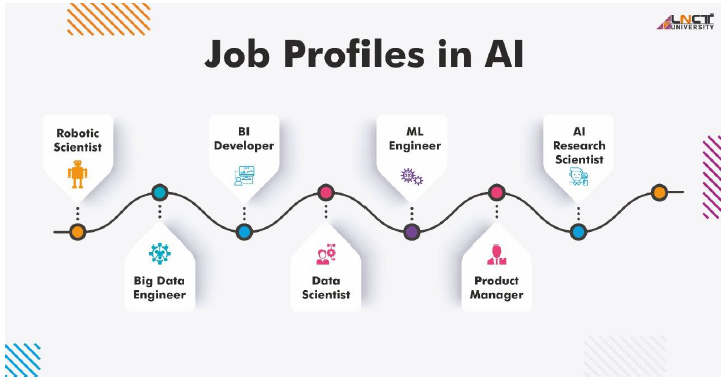
Fig.6 Available at : https://lnctu.ac.in/aiml_careeropportunities/
Regarding the studies related to AI, mostly the background are people coming from STEM subjects such as science, technology, engineering, and mathematics.
There are MOOC available offering great understanding about the subject and few post graduations and master’s degrees.
The person can work for many fields such as healthcare, technology, finance, manufacturing, and logistics companies, looking to develop innovative automation solutions to repetitive tasks.
As mentioning some use cases that companies are doing with AI are:
1-Google is utilizing AI to improve their web search and in the development of autonomous vehicles.
2-Amazon is utilizing AI with the famous Alexa, a chatbot that uses an AI technique called NLP (natural language processing)
3- NVIDIA a known company that develops high-processing hardware to enable faster processing and creation of better AI systems.
4-Apple is utilizing AI with a technique called computer vision that is utilized for facial recognition and is also having a smart assistant called Siri.
4.IoT (Internet of things)
New IoT jobs are emerging such as IOT Engineer, IOT manager and so on.Mostly the background education comes from STEM, especially degrees such as electrical engineering, mechatronics and electronics engineering.
There are some available specializations online with MOOC style, also with universities providing post-graduation and masters programs specifically for IoT knowledge and development.
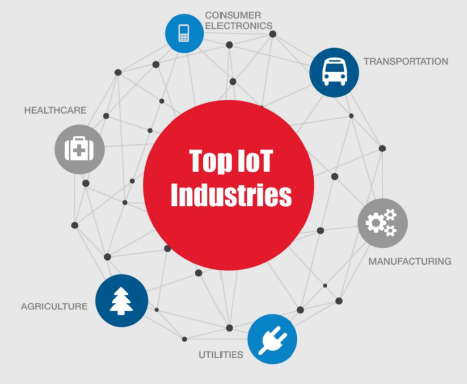
Fig.7 Available at https://www.northeastern.edu/graduate/blog/iot-careers-guide/
Residential and Industrial automation is going to benefit from this emerging technology, it will enable industries to improve and control their process by monitoring in real time the machines with the use IoT sensors while the residential it will enable people to control the temperature or even the security of their home by their phone at any location and time.
Healthcare can benefit from it by monitoring the patients and provide more efficiency in case of emergencies.
Logistics and transportation will have good use of it for monitoring it and having access to localizations in real time.
5.Robotics
Lastly, but not less important, Robotics, which already have known jobs in the market since manufacturing is already having robot arms to automate some of their processes. The most common jobs are Robotics Engineer and Mechatronics.
Therefore, with the improvement of Artificial Intelligence, it will be possible to build more intelligent systems that can solve more repetitive tasks.
Robotics Engineers create and build the physical part of the agent, while AI Specialists have to train and build the programming or the intelligence of it.
Regarding the studies the knowns are the graduations but are having all of its levels and mostly are presence education, anyway there are few online options as well.
ROBOTICS COMPANIES TO KNOW
- Nuro
- CANVAS Technology
- Piaggio Fast Forward
- Diligent Robotics
- Boston Dynamics
- Bluefin Robotics
- Applied Aeronautics
- Left Hand Robotics
- Righthand Robotics
- Drone sense
- Harvest Automation
- Rethink Robotics
- Vicarious
Discussions
This topic aims to share opinions of people abroad related to the emerging technologies described here.
1.Big Data
There are a lot of discussions around the Big Data concept, especially related to its related storage which would be the best infrastructure to store this large amount of data securely while offering accessibility to its content.
There are methodologies to organize this data using Hadoop and its framework that was created specially to deal with Big Data and if this storage should be stored in warehouse infrastructure or data lakes.
Currently there are concerns about how this big data is going to be used by big companies, about data privacy, so there are many questions coming along with emerging technology.
Few challenges concerned about the big data:
1.Privacy – Who owns the personal data and what is made with it.
2.Internet Age – The world has moved to a digital era where any person, company, agency can find people even if they want to or not.
3.Security – as the big data is continuously growing, the data stored is safe and following procedures against data breaches and professional hackers.
4.Safety – what is the level of safety and security related to the data
5.Trust – How trust can be built between safety and security.
6.Ethics – what is allowed and not allowed within the technological age
7.Context – What may be relevant to some users to others it can’t be. How the regulation can approach that.
8.Transparency – Is the companies sharing with transparency about how they are using the user’s data.
2.Data Science
Complementing the evolution of Big Data, there is Data Science, which basically evolved due to the large amount of data available.
New techniques are being developed by research and development centers to keep the technology going.
Assuming the same Big Data issues there are also several other discussions and issues related individually to Data Science.
Data science uses machine learning techniques, which are one of the main techniques of
Artificial Intelligence, eventually several discussions will arise related to both topics, mainly AI.
Machine learning is a technique where the machine is trained to learn how to solve previously defined problems. There are parameters where the machine can decide alone which is the best path or decision to be taken.
In short, the main question is whether these techniques will take the work out of humans and what types of work will be automated, or even if machines will control the world, something that really looks like a science fiction movie, which currently with the technology we have today it is not possible to reach this level of intelligence.
This issue and others will be addressed in more depth in the next topic, which will be about Artificial Intelligence.
3. Artificial Intelligence
Data science benefits from some AI techniques, but AI is at a deeper layer as it involves other techniques like intelligent systems, reinforcement learning and deep learning, these techniques are not very associated with the world of data science.
The main question related to the subject is if these machines will be able to reach the level of intelligence so high, that they will be able to replace humans in their majority of tasks performed nowadays. There is also the issue mainly related to ethics in this situation.
Any subject related to AI generates doubts and fear on the part of people of what can be created with this technology. And although scientists seek to explain what is currently being developed, information has not yet reached the entire society, much of the material is in English and some countries do not speak the language. They are also unaware of the benefits that technology can offer society as a whole.
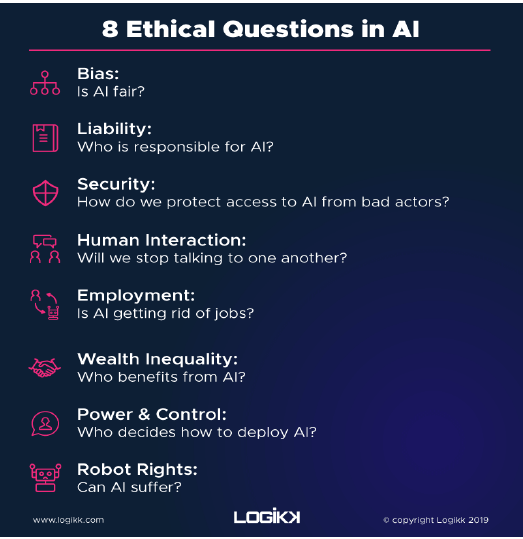
Fig.7 Available at https://www.logikk.com/articles/8-ethical-questions-in-artificial-intelligence/
1) Bias: Is AI fair?
There are key issues when training AI, in fact there are no perfect datasets, so the machine can learn bias related to gender, education, race and wealth. It is important to be considered in AI development to improve bias reduction over time.
2) Liability: Who is responsible for AI?
When an Uber autonomous vehicle killed a pedestrian, it came along with multiple questions such as who is responsible for the technology when things go wrong, is it the developer, the company or even the pedestrian.
People have to be ready for AI system’s mistakes, since humans tend to make mistakes, and I basically is an algorithm made by humans, eventually by time as the technology advances it will do its improvement over time, but still it’s expected to have errors.
3) Security: How do we protect access to AI from bad actors?
Cybersecurity will play an important role in this question, as AI becomes more powerful over time, it will be necessary to develop new methodologies and infrastructure to fight against the bad actors.
4) Human Interaction: Will we stop talking to one another?
The amount of human interaction has decreased, especially after the Covid-19 and the international lockdown applied for many months. As AI continues its improvement, there are rumors that human interaction can decrease each time.
5) Employment: Is AI getting rid of jobs?
This is the main concern between people, it is true that AI will be able to perform some repetitive tasks as humans are currently doing but at the same time it will be creating new jobs, markets and industries as well.
6) Wealth Inequality: Who benefits from AI?
Currently, only big companies are able to invest in AI, so there is a big concern about wealth distribution in the future so that everyone can have a role and participate in the AI economy.
7) Power & Control: Who decides how to deploy AI?
Government will have to create regulations about AI development and operations in the future.
8) Robot Rights: Can AI suffer?
As technology advances, there are questions regarding if AI can be developed to have emotions and how humans have to treat these intelligent machines in the future.
4.IoT (Internet of things)
The two main discussions related to IoT, not only its big potential across many industries and even home automation, but also the main concerns behind the technology, which basically the two main problems are data breach and security.
Main challenges behind IoT infrastructure:
➢ Unpredictable Behavior − as the volume of connected devices increases, it tends to have unpredictable behaviors.
➢ Device Similarity − If one system or device suffers from a vulnerability, many more have the same issue.
➢ Problematic Deployment – the main problem is about how to secure these devices to don’t be easily accessed by third parties.
➢ Long Device Life and Expired Support – One of IoT benefits is the long device life, by the other side, the disadvantage and their systems don’t get support or new updates.
➢ No Upgrade Support − Many IoT devices, like many mobile and small devices, are not designed to allow upgrades or any modifications.
➢ Poor or No Transparency − Many IoT devices fail to provide transparency with regard to their functionality.
➢ No Alerts − Users do not monitor the devices or know when something goes wrong. Security breaches can persist over long periods without detection.
5.Robotics
Basically, when we have a technological approach and have to identify the main concerns and problems, the first defined points are human safety and security, in the robotics world those points are not different.
Robots are getting more advanced and intelligent, especially after the advancement of AI and IoT, the main problems have to be adapted with the new features of it.
➢ Insecure Connections – Most robotic or Iot devices use Bluetooth and Wi-Fi connections, the main problem is the data transmitted is not safely encrypted and there is risk to have access from bad actors.
➢ Privacy Falls by the Wayside – the data is reported remotely to many servers and is available on the web, consequently the data is exposed to suffer attacks.
➢ User Authentication Is Not Strict – the right way to bring safety and minimize risks is by only authorizing users to be able to deliver commands and control it.
➢ Default Configuration Security Is Lacking – configurations, settings, passwords, and account has to be appropriate configured in order to enhance its security and safety.
➢ Enhance Security for Robots – It will be necessary to increase robots’ security as it’s each day becoming more and more part of human lives.
Conclusions
After gathering the knowledge and understanding its cycle, it takes time to believe that this emerging technology is going to be more and more present to our lives and it will mature over time.
Technologies enable people to get more connected and it keeps evolving exponentially in all its sectors not only emerging but as the matured technologies such as cloud computing and ERP.
Some technologies will become obsolete over time as they will be continuously improving.
As can be noticed, one technology pushes the improvement of the other and in the end, it complements each other like a continuous cycle of improvement.
The big data got improved because of the advancement of the hardware. While data science and artificial intelligence got improved because of the Big Data explosion.
IoT gives a great source of data to the Data Science and Artificial Intelligence feed, while robotics can get its improvement from AI and internet of things, and the continuous cycle and so on.
Computer Science World is a challenging field. It requires a lot of effort from the people who decide to dive into this deep ocean of complexity, programming, mathematical models, technologies, and programming languages that are constantly changing.
The world of a computer scientist is full of curiosity and initiative to be always improving within the world of calculations and programming of different languages.
For those who wish to explore these seas, there is great potential to make a difference, especially in the seas of emerging technologies, which will have great relevance for society.
Bibliography
1. Cole Nussbaumer Knaflic ,(2015), “Storytelling with Data: A Data Visualization Guide for Business Professionals”, Wiley
2. Garrett Grolemund, Hadley Wickham, (2015), “R for Data Science”, O`Reilly
3. IBM Artificial Intelligence Engineer Program, Coursera.Org
4. IBM Data Science Specialization Program, Coursera.Org
5. JAMES M. UTTERBACK and HAPPY J. ACEE, (2005), “DISRUPTIVE TECHNOLOGIES: AN EXPANDED VIEW”, International Journal of Innovation Management Vol. 09, No. 01, pp. 1-17
6. Joel Grus, (2015), “Data Science from Scratch”, O`Reilly
7. John J Craig, (2013), “Robotics”, Pearson
8. Kevin Kelly, (2019), “Inevitable”, Alta Books
9. Raji Srinivasan, (2008), “Sources, characteristics and effects of emerging technologies:
10. Research opportunities in innovation”, Journal Elsevier Volume 37, Issue 6, August 2008, Pages 633-64010. Stuart Russel and Peter Norvig, (2020), “Artificial Intelligence – A Modern Approach”, Pearson