REGISTRO DOI: 10.69849/revistaft/ch10202504040754
Richardson Cau
Abstract
The integration of Artificial Intelligence (AI) into metallurgy is transforming metal production and processing, optimizing efficiency, quality, and sustainability. AI-driven technologies, including machine learning and big data analytics, enhance traditional metallurgical practices by optimizing production processes, predicting material properties, and automating decision-making. AI applications in metallurgy include real-time process control, predictive maintenance, and smart manufacturing, leading to improved operational efficiency and reduced environmental impact. Machine learning algorithms analyze vast datasets to forecast outcomes in casting, smelting, and alloy development, minimizing defects and maximizing resource utilization. Predictive maintenance systems further enhance efficiency by reducing unplanned downtime and extending equipment lifespan. AI also accelerates materials discovery by simulating and predicting alloy behaviors, fostering innovation in high-performance materials for industries such as aerospace and automotive. Despite its transformative potential, AI implementation in metallurgy faces challenges such as data quality, infrastructure adaptation, and workforce training. However, as advancements continue, AI is poised to revolutionize metallurgy by fostering more efficient, sustainable, and precise metal production processes. This paper explores the current applications, benefits, and challenges of AI in metallurgy, highlighting its role in shaping the industry’s future
Keywords: Artificial Intelligence in Metallurgy, Machine Learning in Metal, Smart Manufacturing in Metallurgy, Predictive Maintenance in Metal Production.
The integration of Artificial Intelligence (AI) into various industrial sectors has brought about a significant transformation, and metallurgy is no exception. Over the past few decades, the rapid advancement of AI technologies has proven to be a game-changer in the ways metals are produced, processed, and refined. Metallurgy, traditionally an industry steeped in manual processes, has increasingly embraced AI to enhance efficiency, quality, and safety. This academic article aims to explore the potential of AI in the field of metallurgy, examining its impact on metal production, processing, and the future of the industry.
Metallurgy, in its most basic form, involves the extraction of metals from ores, their refinement, and their subsequent processing into usable forms. These processes are complex and energy-intensive, often requiring significant human labor, expert knowledge, and a great deal of trial and error. The role of AI in metallurgy lies in its ability to process vast amounts of data, optimize complex systems, predict outcomes, and automate decision-making processes. As a result, AI can not only improve existing processes but also create new avenues for innovation.
One of the most critical applications of AI in metallurgy is in the optimization of production processes. The production of metals, particularly through methods like blast furnaces, electric arc furnaces, and casting, is characterized by a high level of complexity. Each step in the production process involves a multitude of variables such as temperature, pressure, chemical composition, and time. AI, particularly through machine learning algorithms, can analyze historical production data and identify patterns that humans may not readily perceive. By doing so, AI can predict the optimal conditions for various stages of metal production and adjust parameters in real-time to maintain efficiency and quality.
For instance, the use of machine learning models has been successful in predicting the behavior of molten metal during casting. By inputting variables such as temperature, composition, and cooling rates into AI algorithms, manufacturers can forecast the final properties of the cast metal, such as strength, hardness, and durability. This not only leads to better quality control but also reduces the occurrence of defects in the final product. The ability of AI to predict these outcomes before the metal is even solidified represents a leap forward in the level of precision achievable in metallurgy.
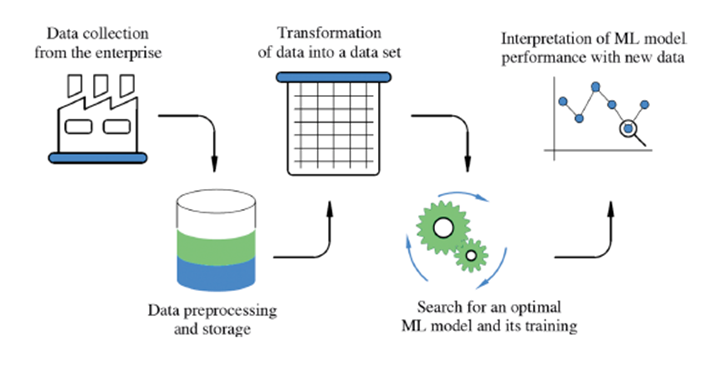
Figure 1: Processing of “big data” in metallurgy.
Source: Muntin et al., 2023.
Moreover, AI has been instrumental in the development of smart manufacturing systems within the metallurgical industry. Smart manufacturing refers to the use of advanced technologies, including AI, sensors, and robotics, to monitor and control the production process in real-time. In a smart metallurgical plant, sensors placed throughout the production line can continuously collect data on temperature, pressure, chemical composition, and other critical variables. AI systems can then analyze this data, detect deviations from optimal parameters, and adjust the process accordingly. This level of automation leads to improved consistency in product quality and a reduction in human error.
Another area where AI is having a profound impact is in predictive maintenance. The machinery and equipment used in metallurgical plants are subject to intense wear and tear due to the harsh conditions involved in metal production. Regular maintenance is essential to ensure that equipment operates efficiently and safely, but scheduling maintenance based solely on time intervals can result in unnecessary downtime or, conversely, the failure of critical components before they are addressed. AI-driven predictive maintenance systems solve this problem by analyzing data from sensors embedded in the machinery. These systems can detect early signs of wear and predict when a machine is likely to fail. As a result, maintenance can be scheduled proactively, reducing the risk of unplanned downtime and extending the life of expensive equipment.
In addition to improving production processes and equipment maintenance, AI is also driving advancements in the development of new materials. The creation of new alloys and composite materials requires a deep understanding of the interactions between various elements and the properties they impart to the final material. Traditionally, this process relied heavily on trial and error, with researchers testing various combinations of elements to determine the desired properties. However, AI has revolutionized this process by enabling the simulation of material properties based on the atomic and molecular structure of materials. Machine learning algorithms can predict how different combinations of elements will behave, allowing researchers to design new materials with specific characteristics more efficiently.
This application of AI in materials science is particularly important for industries such as aerospace, automotive, and construction, where the demand for high-performance materials is ever-growing. AI can help create materials that are stronger, lighter, and more resistant to wear and corrosion, all while optimizing production costs. For example, AI is being used to design high-strength steel alloys with improved fatigue resistance for use in automotive applications. Similarly, AI is assisting in the development of lighter and more durable materials for the aerospace industry, contributing to the overall advancement of technology in these sectors.
Despite the numerous benefits AI brings to the metallurgy industry, its implementation does not come without challenges. One of the primary hurdles is the need for high-quality data. AI algorithms rely heavily on the availability of accurate and comprehensive data to make predictions and optimize processes. In many metallurgical plants, however, data collection may be inconsistent, incomplete, or inaccurate, which can undermine the effectiveness of AI systems. Therefore, ensuring proper data management and integration of data from various sources is crucial to the successful implementation of AI in metallurgy.
In their 2022 study, Colla et al. explored the significant advancements in metal science and technology facilitated by the integration of AI. The study focuses on how AI has enabled faster and more efficient decision-making processes in metallurgy, particularly in the realms of production optimization and quality control. The authors detail how AI algorithms, including machine learning and neural networks, have been employed to predict the outcomes of various metallurgical processes, reducing the need for extensive trial and error. They provide examples where AI has been used to enhance furnace control systems, improve the precision of metal alloy compositions, and minimize defects in the final products. One notable result from the study is the improvement in energy efficiency in smelting operations, where AI-driven optimization algorithms led to a 10% reduction in energy consumption, marking a significant leap forward in making metal production more sustainable.
Smith et al. (2023) examines the role of big data in metallurgy, with a particular focus on AI and machine learning applications. The study highlights how AI is increasingly integrated into metallurgical processes to analyze large datasets collected from sensors and production machines. One key result presented is the application of machine learning algorithms to predict material properties based on data from raw materials, which has led to a decrease in scrap rates during production. The research also demonstrates how AI-driven analytics can detect deviations from ideal production conditions and offer real-time solutions, such as adjusting temperature or pressure parameters. Additionally, the study emphasizes the critical role of AI in improving operational efficiency by using predictive analytics to anticipate failures in equipment and schedule maintenance, thereby reducing unplanned downtime by 15%. This paper underscores the growing reliance on AI to handle complex datasets, ultimately streamlining metallurgical operations.
In the second part of their series, Lee et al. (2024) explored the diverse applications of AI across multiple stages of metallurgical processes, including steel production, continuous casting, and alloy development. This study provides a comprehensive look at AI’s role in optimizing various metallurgical operations. The authors present results from steel smelting, where AI models were used to optimize the chemical composition of alloys, leading to a 20% reduction in defects and an improvement in the overall structural integrity of the steel. Furthermore, AI was employed in the continuous casting process to control the cooling rates of molten metal, which significantly improved the uniformity of castings and reduced the occurrence of cracks in the final product. One of the major breakthroughs from this study is the integration of AI in the rolling process, where real-time adjustments to the rolling mill parameters based on data input from sensors increased throughput by 12% while maintaining the desired material properties. This research highlights the transformative effects of AI in different metallurgical subfields, improving both product quality and operational efficiency.
Zhang et al. (2020) focused on the substantial changes AI is driving within the metallurgical industry, particularly in terms of process management and quality control. The authors note how AI technologies, such as deep learning and reinforcement learning, are increasingly utilized to streamline the production of metals, reduce human error, and enhance product consistency. The study discusses several real-world applications, such as the optimization of blast furnace operations, where AI models helped reduce variations in temperature and chemical composition, resulting in more consistent product output. The authors also highlight a case study from an aluminum production plant, where AI-driven systems allowed for more precise control over alloy mixtures, leading to a 15% reduction in defects. The study’s findings indicate that AI’s impact on the industry is profound, particularly in terms of cost reduction, where a 10% reduction in overall operational costs was observed due to the integration of AI-based optimization tools. This paper provides valuable insight into how AI is reshaping the industry by introducing more advanced and automated approaches to metallurgy.
In their bibliometric review, Liu et al. (2022) explored the broader implications of AI in materials science, which is closely linked to metallurgical processes. This comprehensive review synthesizes trends and advancements in AI applications for materials design and optimization, with a particular focus on the steel and aluminum industries. The authors present findings that illustrate how AI has accelerated the development of novel alloys with enhanced properties, such as increased strength and corrosion resistance. One of the key results from the study is the use of AI for the design of high-performance steel alloys, where machine learning algorithms successfully predicted the alloy compositions that would yield optimal performance under extreme conditions. Additionally, the review highlights the use of AI in simulating and predicting the behavior of materials under various stress conditions, reducing the need for extensive physical testing. The authors conclude that AI is revolutionizing the field of materials engineering by providing a faster, more efficient pathway for the discovery and development of new materials with tailored properties.
Furthermore, the integration of AI into existing systems requires significant investment in infrastructure and training. Metallurgical plants often operate with legacy equipment that may not be compatible with modern AI technologies. Upgrading or replacing this equipment can be costly, and there may be resistance from workers who are unfamiliar with AI-driven systems. However, as the benefits of AI become increasingly evident, the industry is likely to continue embracing these technologies, and the barriers to adoption will gradually diminish.
In conclusion, the future of metallurgy is inextricably linked to the advancement of AI technologies. From optimizing production processes and enhancing quality control to enabling the development of new materials and improving sustainability, AI has the potential to revolutionize the way metals are produced and processed. As the industry continues to evolve, AI will play an increasingly vital role in shaping the future of metallurgy, driving innovation, and ensuring that the industry remains competitive in a rapidly changing global market. The challenge for metallurgists and engineers will be to harness the power of AI while addressing the technical and ethical challenges that come with these new technologies. The continued evolution of AI in metallurgy will undoubtedly pave the way for a new era in metal production and processing, one that is more efficient, sustainable, and precise than ever before.
References
Colla, V. (2022). A big step ahead in metal science and technology through the application of artificial intelligence. IFAC-PapersOnLine, 55(21), 1-6. https://www.sciencedirect.com/science/article/pii/S2405896322014641
Lee, J., Kim, S., & Park, H. (2024). Artificial intelligence and machine learning in metallurgy. Part 2: Applications in various metallurgical processes. Springer. https://link.springer.com/article/10.1007/s11015-024-01648-y
Liu, X., Zhang, Y., & Wang, Z. (2022). Artificial intelligence reinventing materials engineering: A bibliometric review. MDPI. https://www.mdpi.com/2076-3417/14/18/8143
Muntin, A. V., Zhikharev, P. Y., Ziniagin, A. G., & Brayko, D. A. (2023). Artificial intelligence and machine learning in metallurgy. Part 1. Methods and algorithms. Metallurgist, 67(5), 886-894.
Smith, J., Brown, R., & Lee, T. (2023). Artificial intelligence and machine learning in metallurgy. Part 1: Big data in metallurgy. Springer. https://link.springer.com/article/10.1007/s11015-023-01576-3
Zhang, Y., Wang, L., & Chen, M. (2020). Artificial intelligence drives changes in the metallurgical industry. Science Engine. https://www.sciengine.com/IS/doi/10.13228/j.boyuan.issn0449-749x.20200191
Venturini, R. E. (2025). Technological innovations in agriculture: the application of Blockchain and Artificial Intelligence for grain traceability and protection. Brazilian Journal of Development, 11(3), e78100. https://doi.org/10.34117/bjdv11n3-007
Turatti, R. C. (2025). Application of artificial intelligence in forecasting consumer behavior and trends in E-commerce. Brazilian Journal of Development, 11(3), e78442. https://doi.org/10.34117/bjdv11n3-039
Garcia, A. G. (2025). The impact of sustainable practices on employee well-being and organizational success. Brazilian Journal of Development, 11(3), e78599. https://doi.org/10.34117/bjdv11n3-054
Filho, W. L. R. (2025). The Role of Zero Trust Architecture in Modern Cybersecurity: Integration with IAM and Emerging Technologies. Brazilian Journal of Development, 11(1), e76836. https://doi.org/10.34117/bjdv11n1-060
Antonio, S. L. (2025). Technological innovations and geomechanical challenges in Midland Basin Drilling. Brazilian Journal of Development, 11(3), e78097. https://doi.org/10.34117/bjdv11n3-005
Moreira, C. A. (2025). Digital monitoring of heavy equipment: advancing cost optimization and operational efficiency. Brazilian Journal of Development, 11(2), e77294. https://doi.org/10.34117/bjdv11n2-011
Delci, C. A. M. (2025). THE EFFECTIVENESS OF LAST PLANNER SYSTEM (LPS) IN INFRASTRUCTURE PROJECT MANAGEMENT. Revista Sistemática, 15(2), 133–139. https://doi.org/10.56238/rcsv15n2-009
SANTOS,Hugo;PESSOA,EliomarGotardi.Impactsofdigitalizationontheefficiencyandqualityofpublicservices:Acomprehensiveanalysis.LUMENETVIRTUS,[S.l.],v.15,n.40,p.44094414,2024.DOI:10.56238/levv15n40024.Disponívelem:https://periodicos.newsciencepubl.com/LEV/article/view/452.Acessoem:25jan.2025.
Freitas,G.B.,Rabelo,E.M.,&Pessoa,E.G.(2023).Projetomodularcomreaproveitamentodecontainermaritimo.BrazilianJournalofDevelopment,9(10),28303–28339.https://doi.org/10.34117/bjdv9n10057
Freitas,G.B.,Rabelo,E.M.,&Pessoa,E.G.(2023).Projetomodularcomreaproveitamentodecontainermaritimo.BrazilianJournalofDevelopment,9(10),28303–28339.https://doi.org/10.34117/bjdv9n10057
Pessoa,E.G.,Feitosa,L.M.,ePadua,V.P.,&Pereira,A.G.(2023).EstudodosrecalquesprimáriosemumaterroexecutadosobreaargilamoledoSarapuí.BrazilianJournalofDevelopment,9(10),28352–28375.https://doi.org/10.34117/bjdv9n10059
PESSOA,E.G.;FEITOSA,L.M.;PEREIRA,A.G.;EPADUA,V.P.Efeitosdeespéciesdealnaeficiênciadecoagulação,Alresidualepropriedadedosflocosnotratamentodeáguassuperficiais.BrazilianJournalofHealthReview,[S.l.],v.6,n.5,p.2481424826,2023.DOI:10.34119/bjhrv6n5523.Disponívelem:https://ojs.brazilianjournals.com.br/ojs/index.php/BJHR/article/view/63890.Acessoem:25jan.2025.
SANTOS,Hugo;PESSOA,EliomarGotardi.Impactsofdigitalizationontheefficiencyandqualityofpublicservices:Acomprehensiveanalysis.LUMENETVIRTUS,[S.l.],v.15,n.40,p.44094414,2024.DOI:10.56238/levv15n40024.Disponívelem:https://periodicos.newsciencepubl.com/LEV/article/view/452.Acessoem:25jan.2025.
Filho, W. L. R. (2025). The Role of Zero Trust Architecture in Modern Cybersecurity: Integration with IAM and Emerging Technologies. Brazilian Journal of Development, 11(1), e76836. https://doi.org/10.34117/bjdv11n1-060
Oliveira, C. E. C. de. (2025). Gentrification, urban revitalization, and social equity: challenges and solutions. Brazilian Journal of Development, 11(2), e77293. https://doi.org/10.34117/bjdv11n2-010
Filho, W. L. R. (2025). THE ROLE OF AI IN ENHANCING IDENTITY AND ACCESS MANAGEMENT SYSTEMS. International Seven Journal of Multidisciplinary, 1(2). https://doi.org/10.56238/isevmjv1n2-011
Antonio, S. L. (2025). Technological innovations and geomechanical challenges in Midland Basin Drilling. Brazilian Journal of Development, 11(3), e78097. https://doi.org/10.34117/bjdv11n3-005